Triggering reflections and debates in educational contexts
Author:
Greinke, Lena
Citation:
Greinke, L. (2024): Artificial intelligence and photography: Use of AI in educational contexts. In: VisQual Methodbox, URL: https://visqual.leibniz-ifl-projekte.de/methodbox/2024/11/04/artificial-intelligence-and-photography/
Essentials
- Use of Artificial intelligence as a helpful tool for reflection: During discussions with students, AI-assisted imaging can stimulate critical reflections on specific topics.
- Use of Artificial intelligence and photography as a method to enhance creativity: The use of AI to transform photos can help to be creative and think more broadly.
- Artificial intelligence as a support tool: AI can support students, researchers, and practitioners in their research and planning processes (when it is fed with the appropriate prompts and critically reflected).
Description
Admittedly, artificial intelligence (further: AI, and its terminology, such as LLM, AGI, etc.), its impacts for society and science, and its technological black boxes is a maze that many qualitative researchers have not, so far, been able to explore extensively. Whilst utopian and dystopian public narratives compete for framing an AI-future-driven public discourse, many disciplines have started to explore AI’s potential for utilization in research processes, e.g., enhancing quantitative and qualitative data analyses and boosting literature-screening processes. In the following, I will detail a different approach to utilize AI-capabilities for visualization in educational contexts, e.g. to stimulate reflection discussions or to enhance creativity of students in environmental planning.
The historical development of AI goes back to the 1850s. In 1847, for example, a formal language for logic reasoning was described for the first time. AI gained momentum again almost 100 years later when the Turing machine was described in 1936, and the model of artificial neurons was created in 1943. However, the term AI was not used until 1956. Nowadays, however, AI has a different meaning: “creating intelligent agents to help us do our work faster and easier” (Benko & Lányi 2009).
Generating photos with AI is becoming increasingly popular. Often, AIs (such as DALL-E, Stable Diffusion) use web-based interfaces of which many are open source. Various AIs allow users to generate photos by entering prompts, which are textual descriptions of the image. In a course for students of environmental planning, AI was used to do so: In this example, the (in 2022) state-of-the-art text-to-image generator DALL-E 2 was used. Different word combinations and settings can be selected. For example, by entering the same prompt in five different AIs, Göring et al. (2023: 38999-39000) showed that although the AIs can produce images that match the prompts, they do not all look realistic and are of good quality. In some cases, the AI developed somehow realistic flowers, but also created different colors than prompted (e.g. yellow instead of purple) or build artificial or artistic pictures (Göring et al. 2023: 38999).
How the AI works and what the AI changes or reinvents in the photos is usually not explained on the AI websites. It therefore remains a black box as to how the AI reaches its results. Concludingly, it seems problematic to consider AI-images as evidence for something (e.g., the salience of a specific artifact), as the generating algorithms and complexities of information and learning processes in these neural networks resist retrospective dissection. Furthermore, AIs continuously improve with increasing data analysis, user interaction, and increased IT-capabilities.
This introduces us to a peculiar paradox: Despite AI’s increased capabilities, its potentials use cases for data generation have, so far, not shown a comparable inflation. However, I will present one example where AI was used to trigger processes of reflection in an educational context.
Procedure
Depending on the type and use, AI can take on different functions in teaching and learning (Girgensohn et al. 2023: 12f.). “[the] AI model can be your friend, philosopher, and guide … and even your worst enemy” (Chatterjee & Dethlefs 2023). For example, it can be used for inspiration, to concretize a research question or to structure texts as well as for literature research (Girgensohn et al. 2023: 12f.). In order to use AI to stimulate critical reflection by and with students on safety and crime prevention using the example of specific urban areas, different approaches can be applied.
The following example describes a critical reflection discussion on AI and the transformation of photos:
- Step 1: Recruiting motivated students that want to use AI and critically reflect on the results of working with it;
- Step 2: Give an Introduction on a specific topic – in this example it is crime prevention in urban contexts with spatial development and environmental design measures as well as the use of AI with chances and challenges;
- Step 3: Encourage students to research crime prevention measures;
- Step 4: Select a study area that ideally has safe and unsafe places;
- Step 5: Ask the students to take photos (3-5 each) of safe and unsafe places in the study area. If applicable, hand out cameras to take the photos;
- Step 6: If necessary, provide AI software in which the photos taken or generated photos can be changed using prompt inputs. Try out different prompts to transform the photos. Use the previously researched Spatial Development and Environmental Design measures and the background knowledge on AI;
- Step 7: Print out the “most surprising” photos generated by AI and pin them on a board to get an overview of the results;
- Step 8: Firstly, discuss the results in pairs and, secondly, in a larger group. Possible questions for critical reflection could be:
- Which measures could (not) be successfully mapped in the photos?
- How did the AI implement the prompts?
- What influence does the formulation of the prompts have on the results?
- What does the AI need to know/how does it need to be instructed in order to generate “good” results?
- Which AI software is suitable for photo editing?
- What opportunities arise from the use of AI?
- What risks need to be considered when using AI?
- How can we use AI wisely in our (professional) lives, e.g. what do the AI changes say about which aspects make a place safe or unsafe?
- What can we (not) do with the transformed photos?
- Step 9: Summarize the reflection and give some hints what to keep in mind when working with AI and photos.
Requirements
- a scientist (and colleagues) who reflects critically
- if applicable, a (group of) student(s) to engage with
- time to take photos, work with AI and reflect on the results/your work
- if applicable, a camera or smartphone to take photos (if you want to use original/realistic areas)
- an Artificial Intelligence Software to generate photos
Example
In the following, I present an examplary discussion and reflection that I executed with students of the Leibniz University Hannover in the study program B. Sc. Landscape Architecture and Environmental Planning in 2023. Firstly, I introduced the topic and gave a “classic” lecture. I focused in particular on Crime Prevention in urban contexts with spatial development and environmental design measures and discusses the use of AI with potentials and challenges.
Secondly, I introduced a case study – the Raschplatz in the city of Hanover (see Image 1). The Raschplatz is centrally located at Hanover Central Station. Very different uses coincide on the square (train station, restaurants, discotheque, cinema, social facilities, police and many more), which often cause conflicts. Due to its proximity to the train station and other social facilities located there, the Raschplatz is also often a place where socially disadvantaged people and people at risk of addiction spend time. In the past, various interim uses in the form of sports and cultural events have already been tried out in the area. There have already been numerous discussions in the media about safety on and around the square, which have given it a rather negative image. Consequently, this urban square, which is the subject of multilayered conflictual discussions in the city, is very suitable for analyzing safe and unsafe place in connection with crime prevention. The aim is to analyze to what extent crime prevention measures can contribute to ensuring that places become safer and to what extent AI can support the process of developing and reflecting these measures.
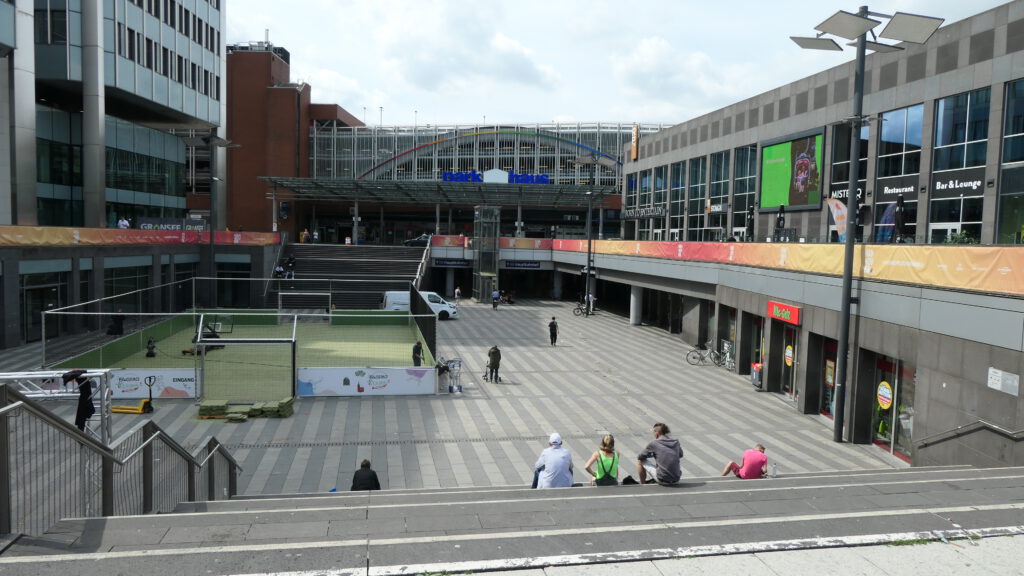
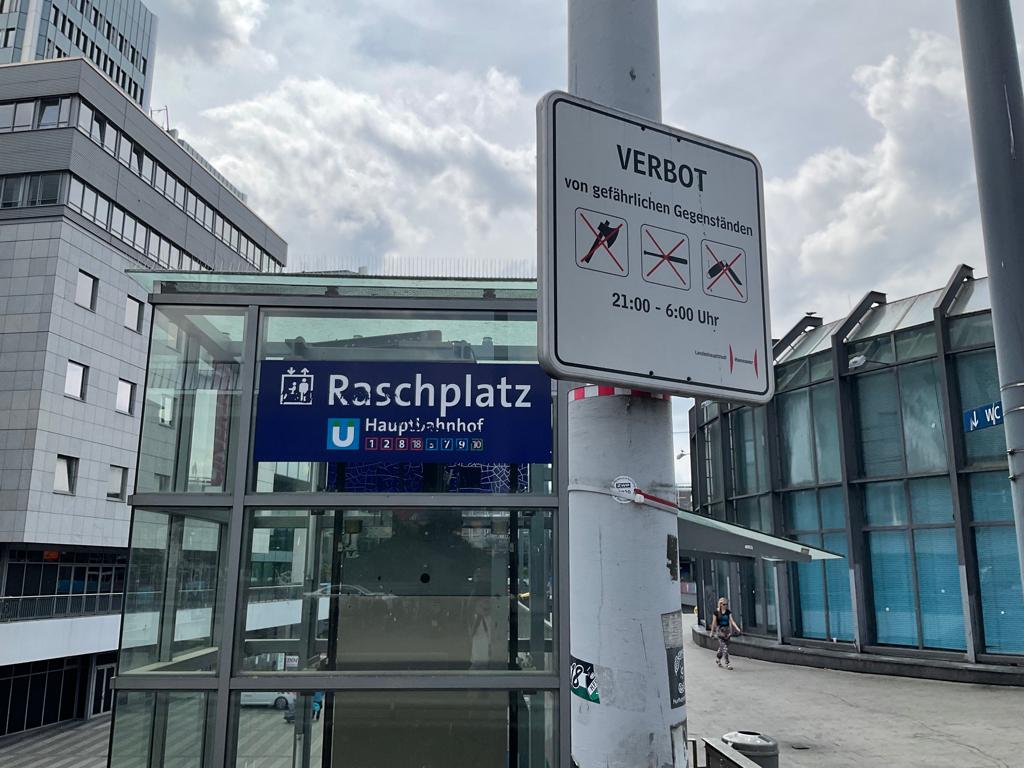
Image 1: The case study “Raschplatz” in the city of Hanover (Source: Amelie Streit & Marlene Reinmüller (Abb 1_1); Robin Mudrack (Abb 1_2))
Thirdly, I decided that the students should not just execute a desk research on my input and summarize crime prevention measures they observed. I asked the students to take photos (3-5 each) of safe and unsafe places in the study area (see Image 2). They used their own cameras or smartphones to do so.
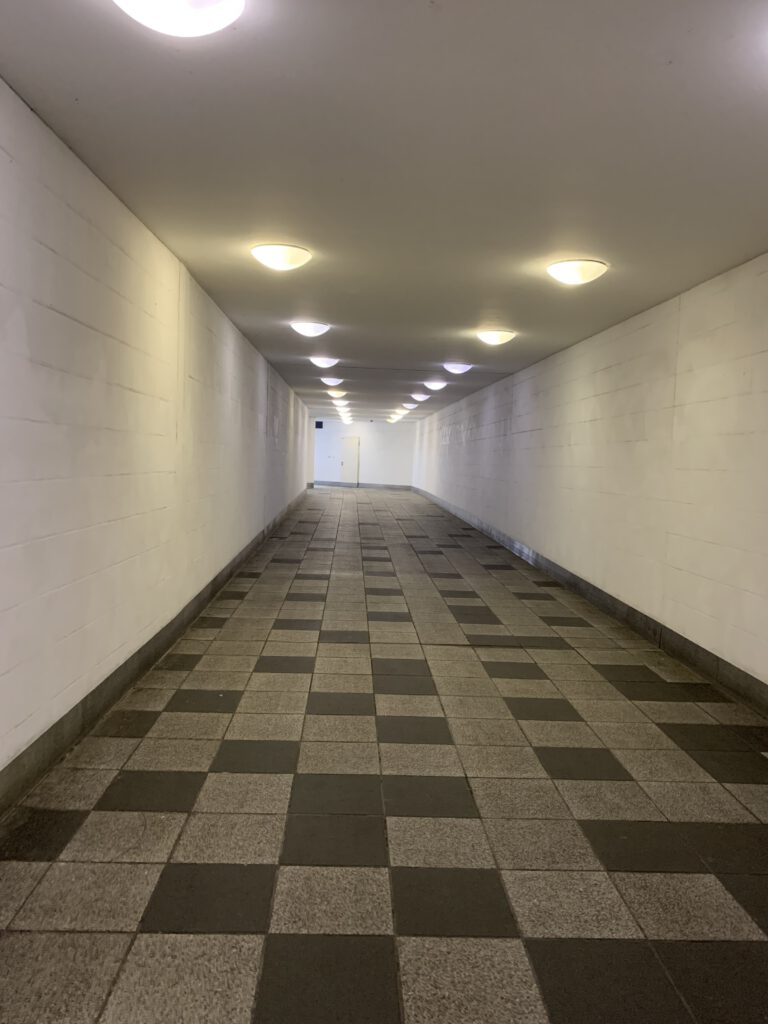
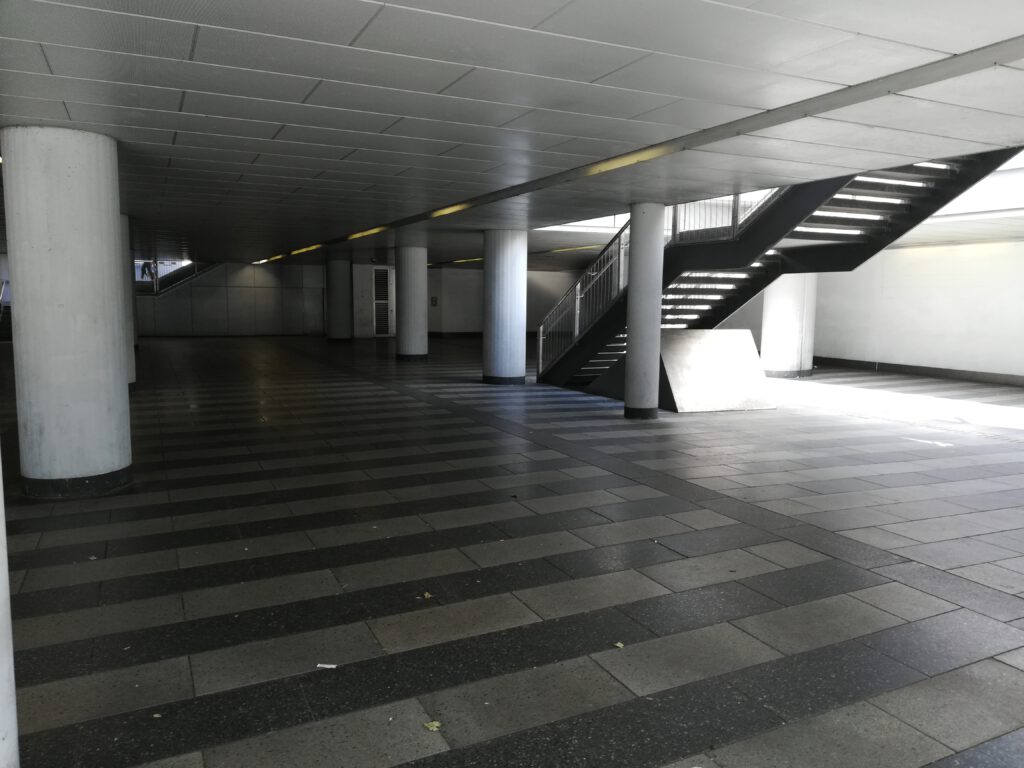
Image 2: Photos of safe and unsafe places from the students (Source: Svea Grothstück (Abb 2_1); Mareike Müller (Abb 2_2))
Fourthly, the photos were uploaded into the AI software Dall-E 2. Dall-E 2 is an AI that creates or changes realistic images and art from prompts. The software was given the instruction to change the photos using the prompts from the students. For example, they try to “safer” the place through environmental design using prompts like “more light” or “more greenery”. The students also tried to generate new photos with the AI. The students tried out different prompts to transform the photos using their previously developed spatial development and environmental design measures (see Image 3, 4 and 5).
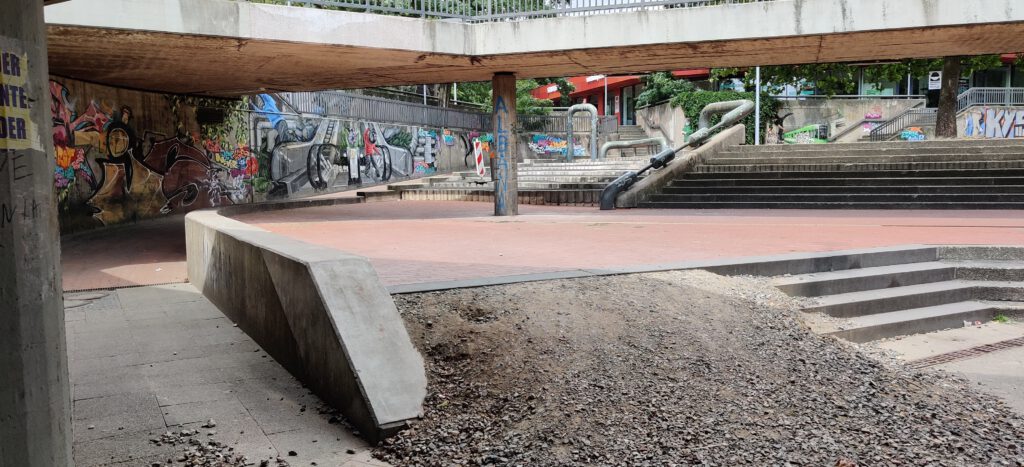
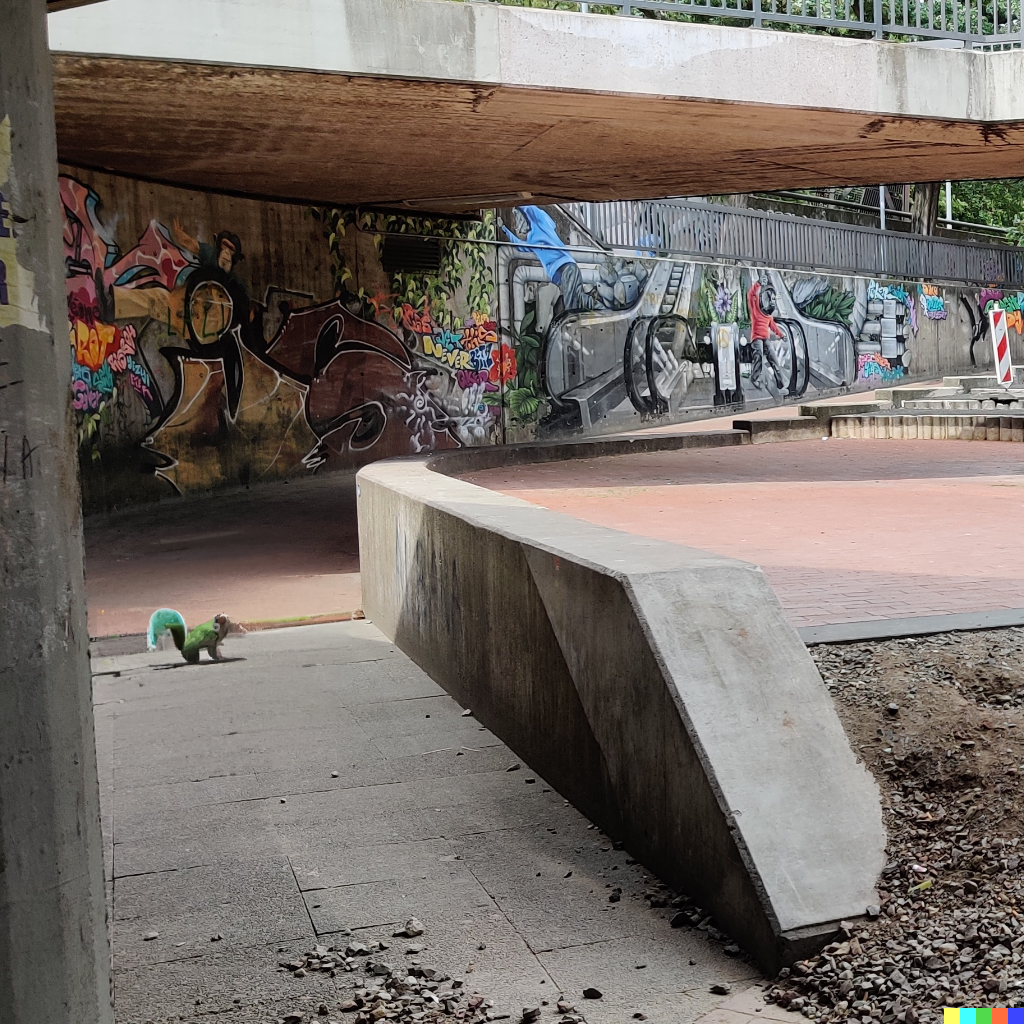
Image 3: Examples of the transformation with the AI “Dall-E 2” (left original, right transformation) (Source: Keno Lou Freiholz (Abb 3_1); Dall-E 2 (Abb 3_2))
Lastly, the students chose the “most surprising” photos generated by AI, printed and pinned them on a board in the class room. With this collection, we all got an overview of the results and started the discussion. Therefore, the students first presented their results in form of a poster with the surprising photos in pairs and afterwards in the whole group. They described their procedure of developing and changing photos as well as their challenges during their work. The aim was to sensitize for the work of the AI in a black box, but also to critically reflect on their behavior while using AI for their professional work. We critically reflected on different questions (see Procedure above) and clustered the photos into different categories. The students were very motivated and talked intensively about the challenges and opportunities of AI. In the end, I summarized the reflection and gave some hints on what to keep in mind when working with AI and photos.
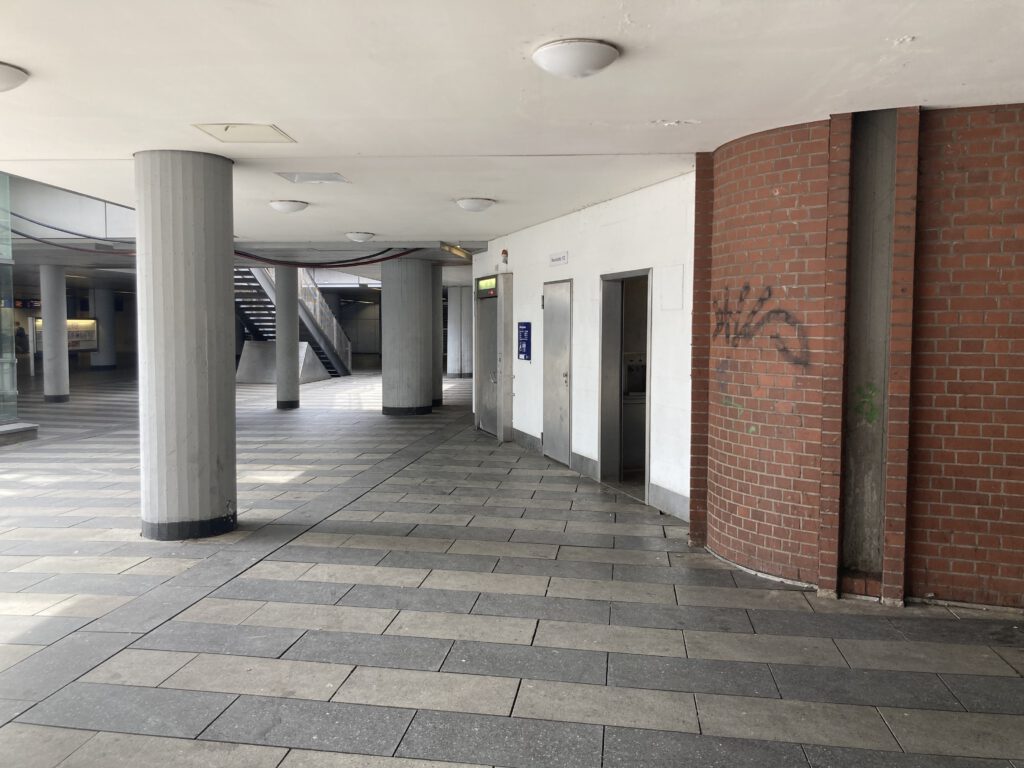
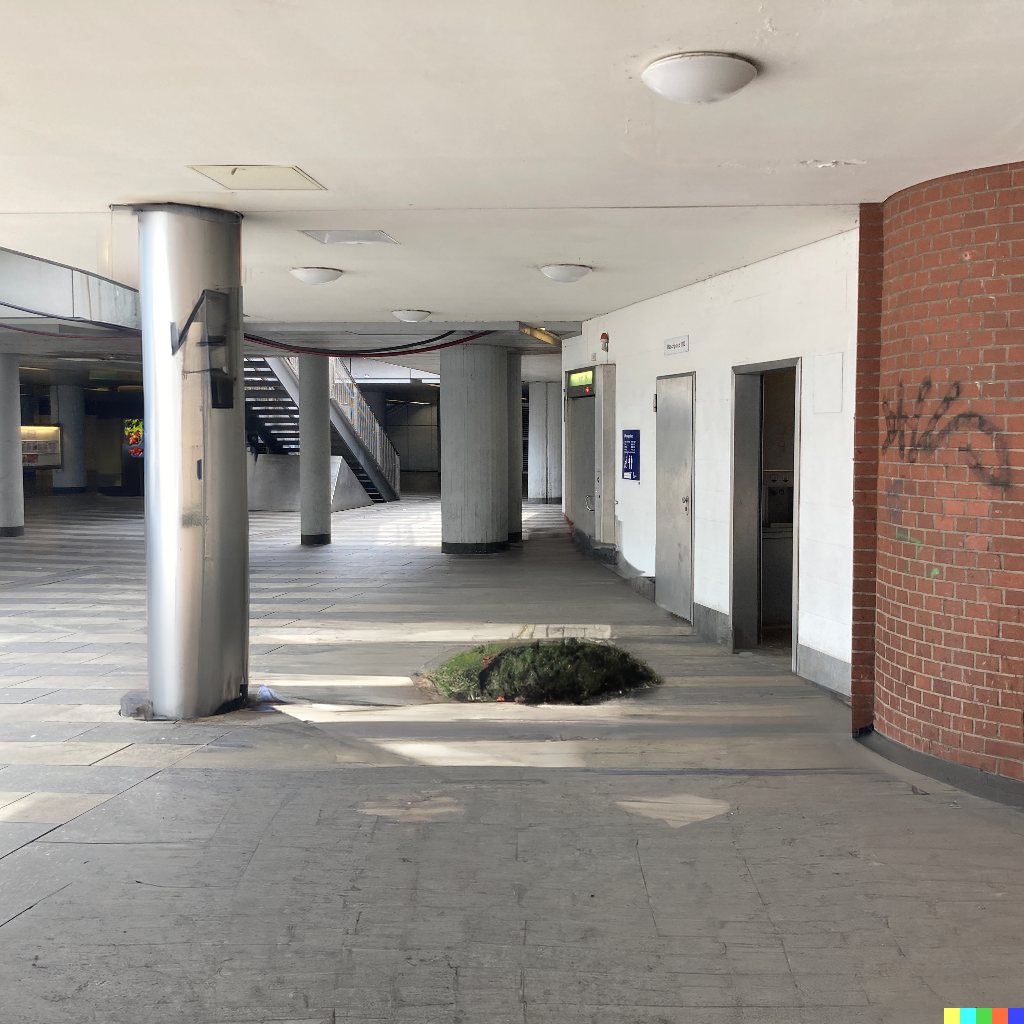
Image 4: Examples of the transformation with the AI “Dall-E 2” (left original, right transformation) (Source: Nemo Sonko (Abb 4_1); Dall-E 2 (Abb 4_2))
Overall, the students were very interested in working with AI. However, the follow-up reflection also encouraged them to think deeply. This led them to critically discuss their work and to frame the results properly. As one can see in images 3, 4 and 5 show, for example, is that the AI places a fictitious squirrel in the path (image 3), establishes green spaces in front of entrance doors (image 4) or creates more light and greenery by enlarging ceiling holes with hanging plants. AI can therefore implement the principle of more greenery and more lighting, but does not integrate this into the spatial context (Greinke et al. 2023: 30). AI often supplements building changes, such as planting, lighting or safety signs, but excludes social aspects. However, these aspects are eminently important for an increased sense of safety (Greinke et al. 2023: 31). From the modeling with AI, the students reflected, for example, that AI can provide ideas, but is rather poor at placing them spatially. It is also important to pay attention to the “appropriate” use of the prompts so that they can be implemented by the AI. For planners, AI can therefore certainly provide support, but the results should always be critically evaluated (Greinke et al. 2023: 31). More critical reflection on this method can be found in the following publication: Greinke, L., Richter, J. S., & Lange, L. (2024). Kriminalprävention durch Künstliche Intelligenz: Kritische Reflexion am Beispiel des Raschplatzes, Hannover. Planerin, 2024(4), 29-31.
The effort involved in using AI compared to photo documentation and image analysis is not less, but probably not significantly greater either. The generated images must be analyzed and critically reflected upon in the same way as would normally be required for image analysis. However, the step of generating suitable images can take more time, as this is not necessary with photo documentation and image analysis. It can be expected that AI will continue to learn and that image generation may therefore become even better or more suitable and faster in the future.
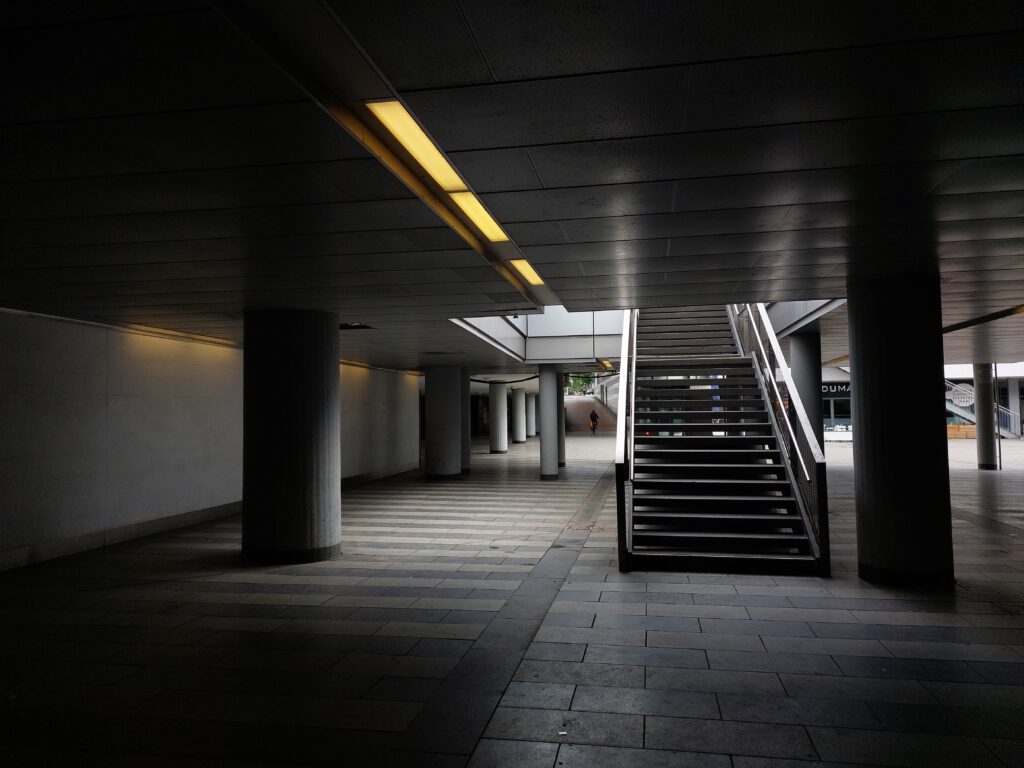
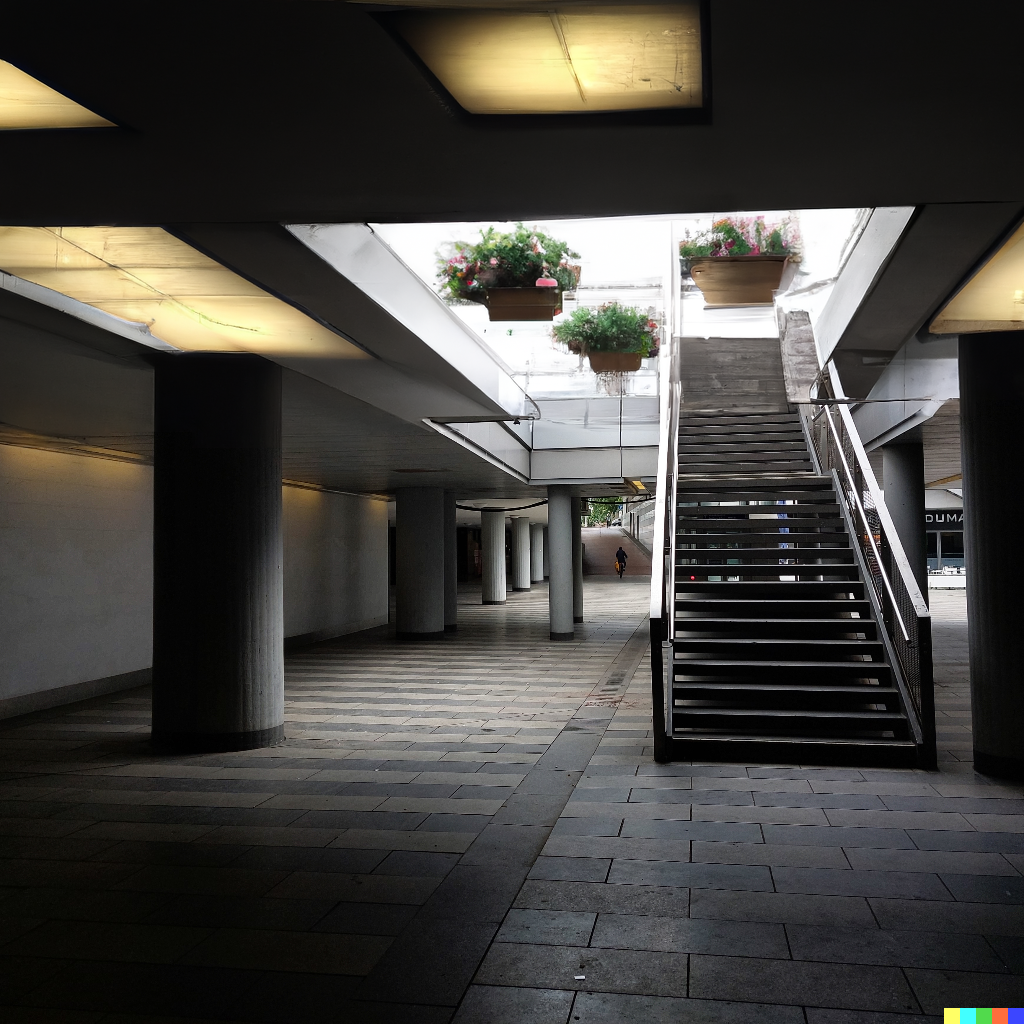
Image 5: Examples of the transformation with the AI “Dall-E 2” (left original, right transformation) (Source: Johanna Weißflog (Abb 5_1); Dall-E 2 (Abb 5_2))
Useful Resources
- Artificial intelligence for creating photography, e.g. Dall-E 2 (https://openai.com/dall-e-2/); Midjourney (https://www.midjourney.com/); Stable Diffusion (https://huggingface.co/spaces/stabilityai/stable-diffusion); Glide (https://www.glideapps.com/); Craiyon (https://www.craiyon.com/)
Suggested Tools
- Camera or smartphone
- Artificial intelligence Software, e.g. Dall-E 2
- Room with a board to collect the result (possible in presence or virtually)
- If analogue: Printer to print the transformed photos, moderation materials with sticky notes and pens etc. to cluster the results
References
Benko, A. and Lányi, C. S. (2009): History of Artificial Intelligence. In: Encyclopedia of Information Science and Technology. https://doi.org/10.4018/978-1-60566-026-4.ch276
Chatterjee, J. and Dethlefs, N. (2023): This new conversational AI model can be your friend, philosopher, and guide … and even your worst enemy. In: Patterns (New York, N.Y.) 4 (1), S. 100676. https://doi.org/10.1016/j.patter.2022.100676.
Girgensohn, K.; Mundorf, M.; Gholiagha, S.; Voigt, J.; Fröhlich, B.; Kiesel, D.; Neyer, J.; López García, I.; Riehmann, P.; Sienknecht, M.; Stein, B.; Wiegmann, M.; Wolska, M. A. (2023): Forschendes Lernen mit KI im Sozialwissenschaftlichen. KI-Labor für Forschendes Lernen (SKILL). Konzeptionspapier Hochschuldidaktik. Frankfurt (Oder), Słubice und Weimar.
Göring, S.; Ramachandra Rao, R. R.; Merten, R. and Raake, A. (2023): Analysis of Appeal for Realistic AI-Generated Photos. IEEE Access, Volume 11.
Greinke, L., Richter, J. S., & Lange, L. (2024). Kriminalprävention durch Künstliche Intelligenz: Kritische Reflexion am Beispiel des Raschplatzes, Hannover. Planerin, 2024(4), 29-31.
Leave a Reply